Jonathan Bosch, Cam Mitchell, Drew DiSanto, Dominic Samangy &
Syracuse Basketball Analytics Club Presents
Does College Experience Affect NBA Success?
Background:
The game of basketball is changing before our eyes. It is shifting to a fast-paced, three point shooting, jaw-dropping dunk-filled sport. Along with these on-court changes, there has been another trend developing within the NBA in regards to drafting. The NBA has most notably become infiltrated, and arguably dominated, by young stars. NCAA athletes are forgoing their remaining years of eligibility at a higher rate to chase their professional aspirations. NBA teams are drafting younger players with the expectation that they can train them to reach their maximum potential. With this great influx of new talent available in the draft, scouts have been tasked with the difficult job of evaluating raw and underdeveloped talents they have not really seen before. This has led to the question we will look to answer in this study. Does College Experience Affect NBA Success?
Data:
In order to best answer the question at hand, we utilized public data, scraped from basketball-reference.com. The data for the players includes information from their collegiate and NBA careers from the 2006-2015 seasons. Listed below are the variables and metrics considered in our analysis.
Player Name, College Attended & Year Drafted
These variables are self-explanatory and necessary to build an accurate sample of picks. They also allow for easier manipulation in terms of filtering, cleaning, or arranging the data. For this sample, only draftees that attended an NCAA Division 1 institution were included.
Draft Pick # (Pk)
This variable is extremely important to the study as it allows us to classify and organize all the draft picks into four categories: lottery picks, late 1st round, early 2nd round, and late 2nd round. This is the basis of our study and defines how we are going to determine where the most valuable picks in the drafts are most commonly chosen.
College Experience
Like draft pick #, college experience is essential to determine where value lies in the draft based on experience. The dataset includes players who have 1-4 years of on-court experiences, thus leaving out grad-transfers.
Years Played in NBA (Exp)
Years played in the NBA allows us to quantify success based on draft picks. In order to do so, we have included the first four years of data for each draft pick as this mimics the standard length of a rookie contract. This enables us to most accurately quantify the value that an organization received for their draft pick.
NBA Win Shares (success1, success2, success3, success4, wsTotal)
In order to quantify success in the NBA, win shares are used as our determinant. According to basketball-reference, “win shares is a player statistic which attempts to divvy up credit for team success to the individuals on the team.”
Methods:
In total, our method of analysis included utilizing basketball-reference.com, google sheets, and RStudio. The initial retrieval included a manual scrape of the website as data was then organized by draft class and eventually into a master Google sheet. After retrieving the necessary data, we turned our attention to utilizing RStudio software to clean and filter the information to ensure the most accurate analysis.
For the analysis, each range of draft picks (as described above) will have a table with NBA experience on the left side column and NBA success across the top row. One should read each success column as the expected success of a draft pick picked in that range for that specific experience. The success of that pick is the mean of the win shares of all picks for their respective seasons. Success1 represents their rookie season, success2 being sophomore season, and so on. For example, based on the first table below, we expect, on average, a one-and-done lottery pick to produce a win share of 2.232 in their first NBA season. This would equate to the highest expected success for a draft pick, therefore providing the most value.
Analysis & Results:
Lottery Picks (#1-14)
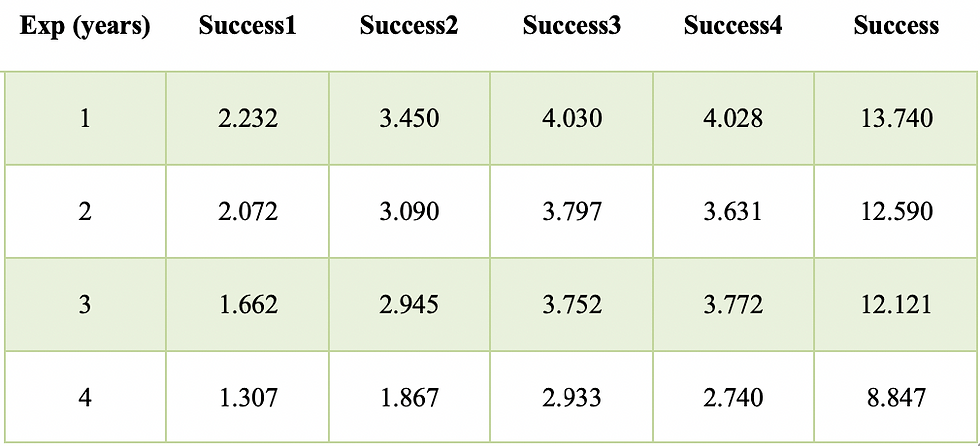
As briefly discussed above, for lottery picks, our results show that a one-and-done lottery pick has out produced the other years of experience. With a total expected win share statistic of 13.740 for their rookie contract, we can infer that recent high level one-and-done D1 players are the most valuable draftees for NBA organizations. It is interesting to note that success drops significantly for players with four years of collegiate experience. With a value of approximately 8.847 wins, these players should be avoided at this level and should be targeted potentially in the late 1st round.
Late 1st Round (#15-30)
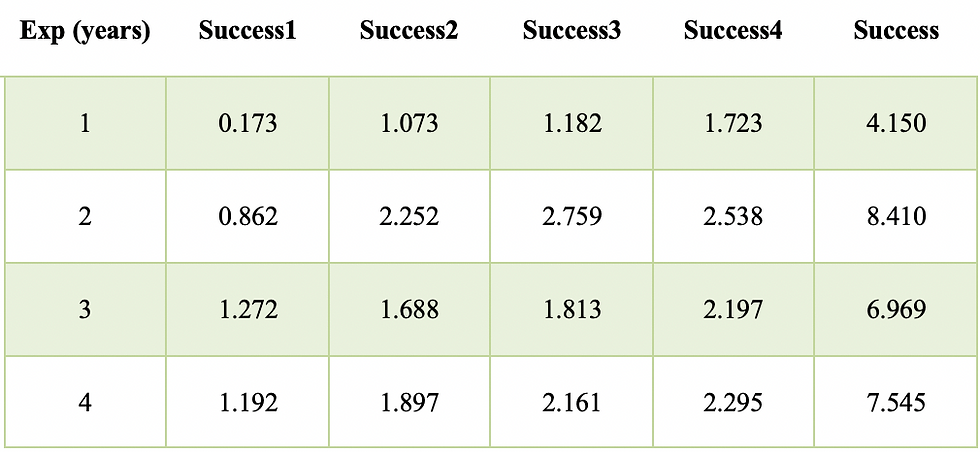
For late 1st rounders, the trend is distinctly different. Players with two years of experience had the greatest amount of total success over their rookie contract, despite contributing the least during their rookie season. Therefore, while they may take less time to develop, they appear to be the most profitable in the long run. In contrast to lottery picks, one-and-done players provide by far the least amount of value in the late 1st round with a WS total of 4.15. With all this information, this draft range seems to be filled with developed and established players or late 1st round gambles taken on young, raw, but potential-filled prospects.
Early 2nd Round (#31-45)
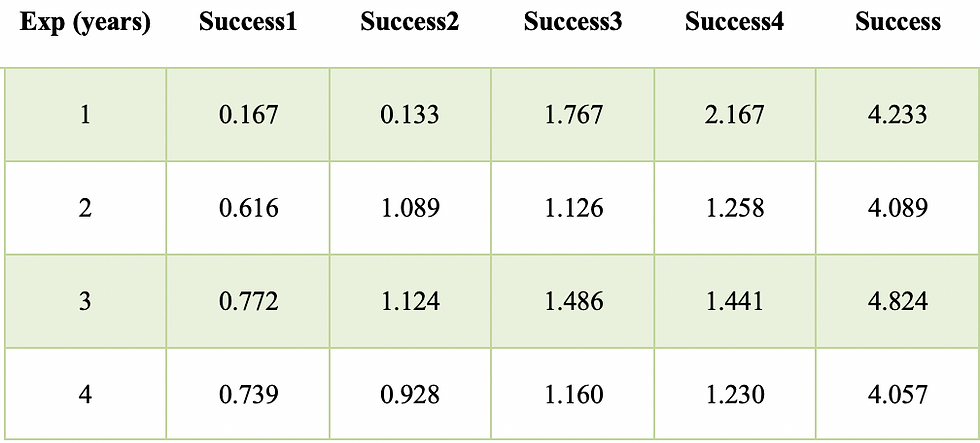
In the early 2nd round, one-and-done players perform worse in their first two seasons but then produce the most over the course of the next two. However, over the course of the four seasons, players produce very similarly regardless of collegiate experience. Therefore, it is important for teams to look at this based on their team’s current competitive status. For teams looking for an immediate impact, it would make sense to look for an experienced and developed prospect. On the other hand, for a team building for the future, it might be most beneficial to select a one-and-done prospect with the hopes of them fulfilling and potentially exceeding their raw potential.
Late 2nd Round (#46-60)
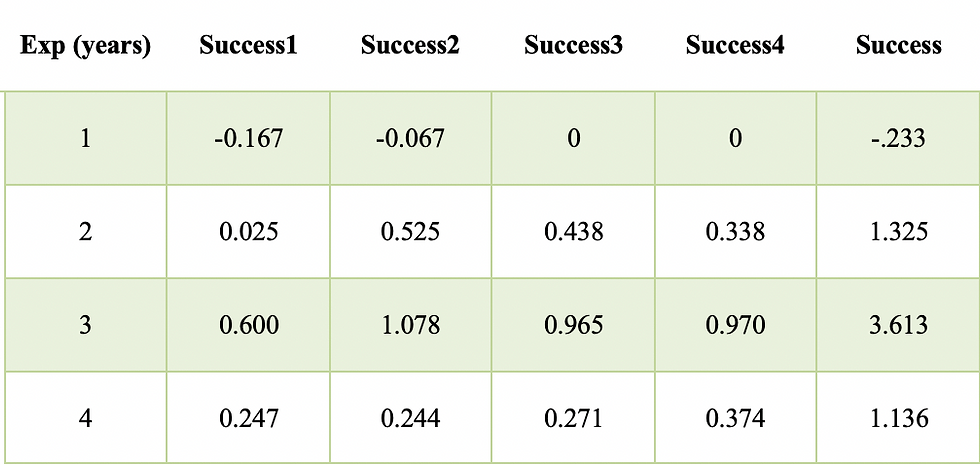
The trend seen in early 2nd round picks continues into the late 2nd round territory but at a much more drastic and evident rate. One-and-done players contribute at a shocking rate with no positive expected win share values over their four years and a negative total success statistic. In contrast, the other three experience samples performed much better. It is also evident that players with three years of experience performed at the highest rate with an expected total win shares of nearly three times that of players with two or four years of experience. In review, teams should look to add bench value with experienced players in this range with the hope of them carving out a bigger role towards the end of their rookie contract.
Implications:
Although this study includes an accurate sample, there are a couple limitations. First, the research only takes on-court experience into account, leaving out relative experience such as a red-shirt season or a sit-out transfer season. For example, Elijah Hughes of Syracuse has recently declared for the 2020 draft as a red-shirt junior and is predicted to be a second-round pick. In the prospective 2020 dataset, he would fall under 3 years of collegiate experience. However, he experienced four years of basketball experience and practicing although he only played games in three seasons. Therefore, although unnoticed in the final dataset, he would have an advantage on draftees who had only three years of both on-court and relative experience.
Second, upon further investigation, there will be some outliers found that do not adhere to any of the trends in the aforementioned tables. For example, Brandon Roy, who played four seasons at the University of Washington, was selected with a lottery pick at #6 in the 2006 NBA Draft. According to our study, he would contribute an expected 8.85 win shares during his first four seasons. However, he amassed a win share total of 35.4, exactly 5 times above his expected value. On the other hand, Xavier Henry was a one-and-done lottery pick in 2010 who totaled a win shares value of 0.7 in his first four NBA seasons, far less than the expected 13.74 statistic.
Lastly, because this study focuses on utilizing college experience to predict NBA success, the sample does not include international draft picks or non-D1 players. If needed, a future analysis could possibly incorporate each of these, thus providing a more in-depth study.
Conclusion:
As covered in the implications section, the expected values of success provided does not guarantee success or failure for specific draft picks. There will undoubtedly be players who over-perform and under-perform. Therefore, this study should not be the all-conclusive factor for NBA scouts and front offices when evaluating talent. However, like with many other pieces of information, either quantified or observed, it can help guide and encourage better-formed decisions.
Comments