A K-Means Clustering Approach to NBA Draft Big Boards
- Dominic Samangy
- Aug 25, 2021
- 8 min read
Dominic Samangy // @DSamangy
NBA Draft big boards are an extremely popular exercise where an evaluator ranks each prospect in a numerical order based on their player assessment opinions and philosophical beliefs. Whether they value certain on-court tendencies differently or place more weight on other factors such as age and “fundamentals”, the rankings are reported in a one-dimensional manner. While this exercise can be beneficial, it seems highly inefficient to gauge each prospect on the same benchmarks and to then rank them all at once in numerical order. Another common form of a big board that somewhat solves this issue, is to rank players based on their positions (PG, SG, SF, PF, C). Positional labels such as these do their job for box score reports but fall way short in terms of in-depth prospect evaluations and projections.
Rarely do any two players contribute in the same manner, but instead are all dissimilar and can provide value in unique ways. Therefore, we need to do a better job at assessing these prospects for what they are and not for what a box score describes them as. Thus, the goal of this study is to analyze the recent 2021 NBA draft class through a statistical clustering technique that will provide a player archetype-focused big board. Not only will this give us a better look at what each player brings to the table but will also allow us to rank players based on their respective roles instead of all at once.
Data
The data collected for this study includes physical measurements and performance statistics on the top 150 prospects in the 2021 NBA Draft according to Rookie Scale’s weighted average. The statistics for collegiate and G-League players were pulled from basketball-reference.com while all international data was pulled from basketball.realgm.com. The variables included in the cluster analysis are listed below:
Physical Measurements
- Height & Weight
Performance Statistics
- PTS/40, 2PA/40, 3PA/40, FTA/40
- 2P%, 3P%, FT%,
- AST/40, TOV/40
- STL/40, BLK/40, ORB/40, DRB/40, PF/40
Methodology
For this study, I will utilize a K-Means technique, which is one of the most popular clustering approaches. K-Means is an unsupervised clustering algorithm that requires no input of a response variable. This is because the goal of the model is not to be predictive but instead to identify similarities between the observations of a dataset. Using the Euclidean distance between each observation, these groupings are produced with the goal of identifying observations (players) that closely relate to one another. In shorter terms, this model will randomly look to identify players that measure & perform similarly based on their height, weight, and their performance statistics before placing them in clusters, or groupings. After doing so, we will be able to draw our basketball-related conclusions, such as labeling archetypes of each cluster and ranking each player in their respective groupings.
When examined, the dataset in question suggests the optimal number of clusters to specify for the model is 10, which will ensure that there is a maximum distance between clusters and minimal distance within the clusters. The goal of this study isn’t to focus on statistical methodologies but instead on creating an interesting alternative to the traditional big board and its importance to draft discourse. Therefore, instead of going further into the practice of clustering, I’ll attach a link below with a link to a further explanation if you are interested: https://www.datanovia.com/en/lessons/k-means-clustering-in-r-algorith-and-practical-examples/.
Clusters
Finally, with the data collection and clustering performed, we can look to analyze the model’s results. The roles assigned are based on my analysis of the cluster and their statistical strengths and weaknesses. First up, cluster 1:
Cluster 1 -- Shot-Blocking Bigs
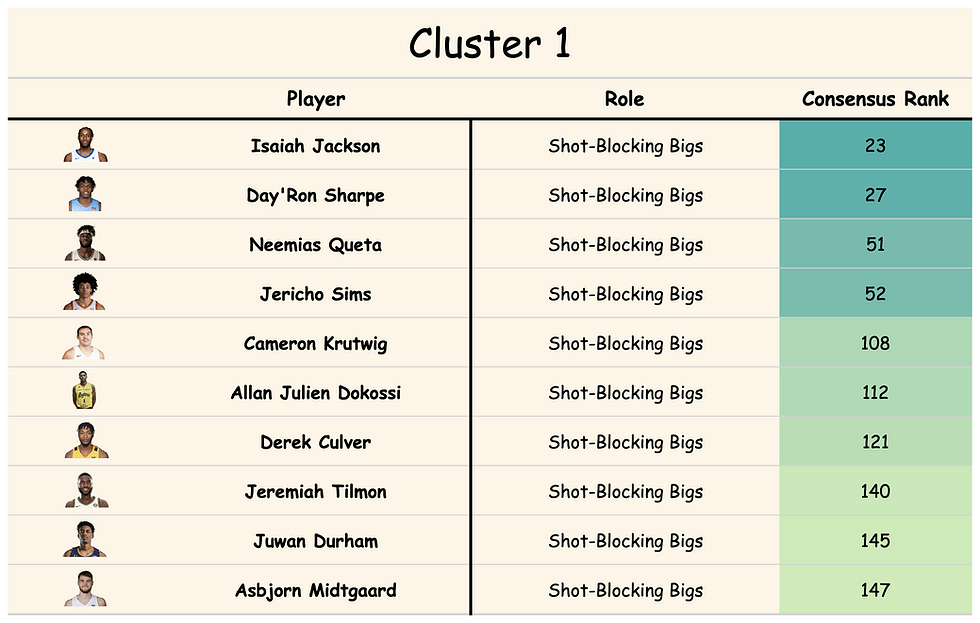
- Strengths
o ORB/40, Weight, BLK/40, DRB/40, Height
- Weaknesses
o 3P%, 3PA/40, FT%
The first cluster certainly doesn’t disappoint. From Isaiah Jackson to Jericho Sims, these guys are your interior forces in which the paint is their bread and butter. They’ll fill up the stat sheet with blocks and rebounds while primarily only scoring within an approximate 8-foot radius. It is interesting to note that the consensus ranking of this cluster coincides with how the game is shifting. While there will always be a need for rim protection, if a prospect of this mold doesn’t bring much else to the table, they may not be eating as well as one would in the past.
Cluster 2 -- Shooting/Slashing Wings
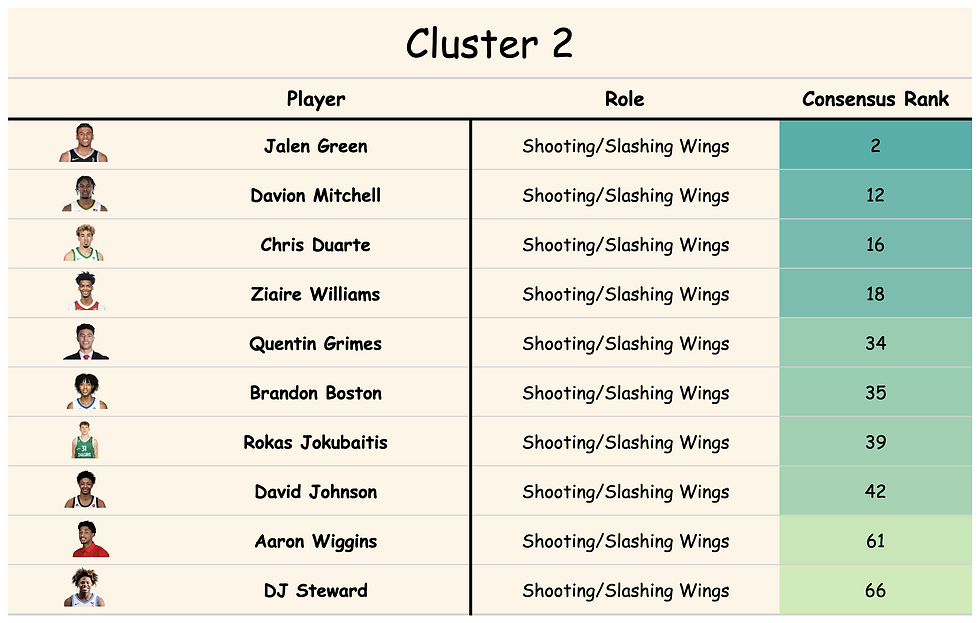
- Strengths
o 3PA/40, FT%, 3P%, STL/40, AST/40
- Weaknesses
o Weight, DRB/40, ORB/40, FTA/40, Weight
Next up, we have the notably lightweight guards/wings that can fill it up from long-
range. While they don’t test off the charts in terms of scoring, a few names in the cluster might suggest otherwise. Perhaps a front office would like to bet on both? This group can also contribute as secondary ball-handlers and will more than likely be serviceable on the defensive end.
Cluster 3 -- Low Usage Stretch Bigs
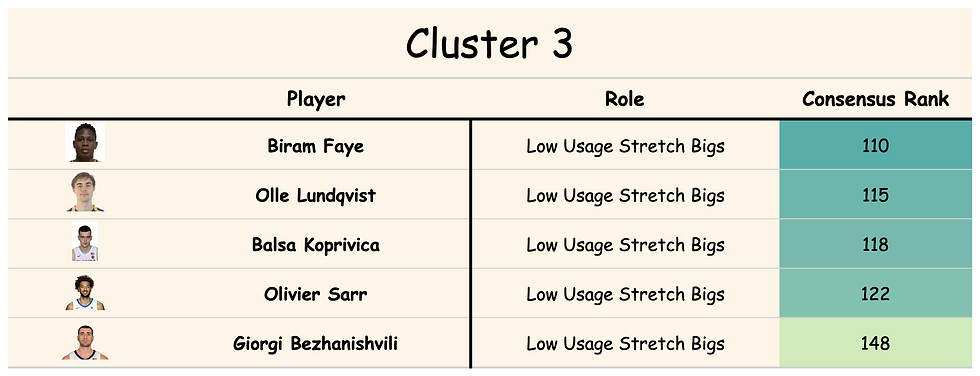
- Strengths
o 3P%, Height, ORB/40, Weight
- Weaknesses
o PF/40, TOV/40, STL/40, 3PA/40
Third, we have quite an odd group of names that I was not expecting at all. These 5 prospects are rebound heavy bigs who are above average in 3P%, despite a couple of them rarely taking threes. The assignment of a cluster for these guys may be more down to what they don’t bring to the table instead of what they do in comparison to other forwards/bigs prospects. Nevertheless, results such as these with this clustering approach are what make us ask different questions about our evaluation tendencies, and that’s all we can ever ask for.
Cluster 4 -- Oversized Modern 4s
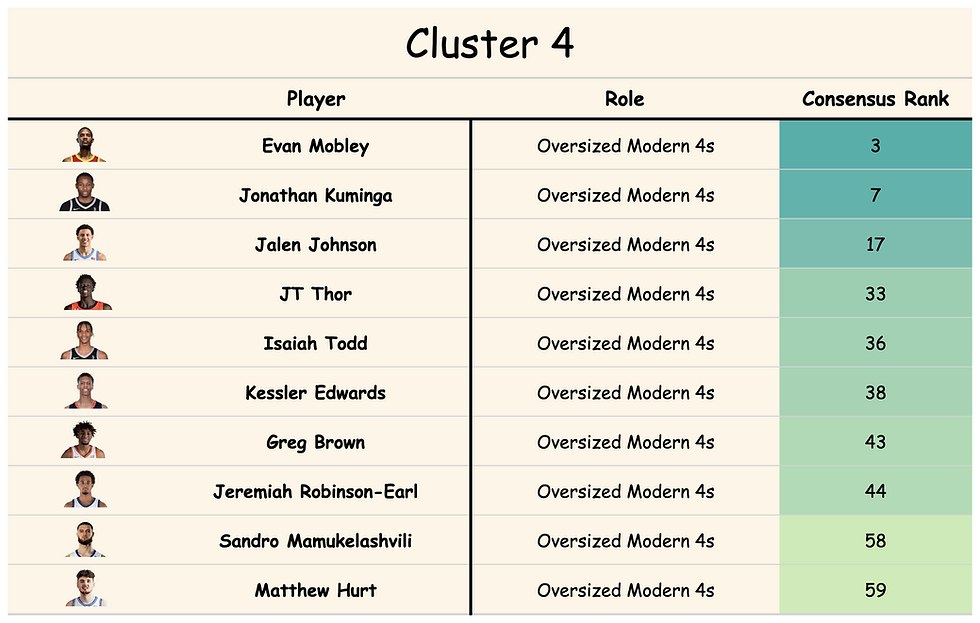
- Strengths
o Height, BLK/40, Weight, DRB/40, ORB/40
- Weaknesses
o AST/40, STL/40, 3PA/40
Cluster 4 is evidence of how the modern game has evolved. These are the prospects that arguably draw the most divisive opinions in terms of both their immediate and future value to a team. Players such as Evan Mobley and J.T. Thor are examples of the “unicorn” label that pops up way too often. However, despite the disparity between draft evaluators, they certainly never fail to entertain us on the court. The high-flying dunks and blocks mixed with the self-creation and 3-point shooting flashes are what attract NBA scouts and the average consensus ranking of 33.8, the 2nd highest of any cluster, shows that the media is right there with them.
Cluster 5 -- Defensively Astute Ball-Handlers
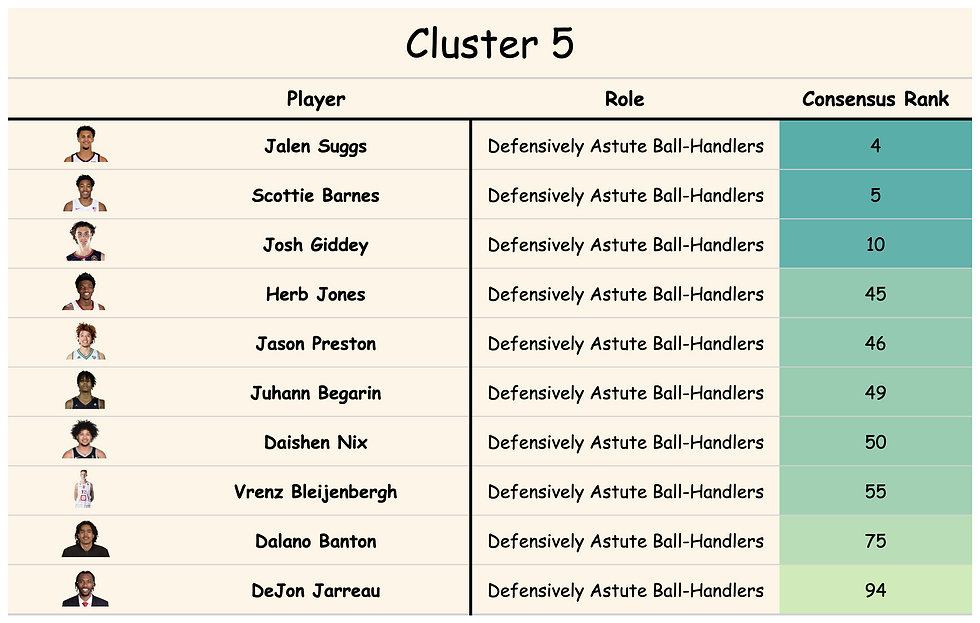
- Strengths
o AST/40, STL/40, DRB/40, Height
- Weaknesses
o FT%, PTS/40, FG/40, FT/40
In cluster 5 we run into our ball-stoppers. This group is known for their defensive
reputations and players such Jalen Suggs, Scottie Barnes, Herb Jones, and DeJon Jarreau are the epitome of such. While they draw a lot of attention on that side of the court, they fit the ball-handling role just as well. Yes, some of the names may not pop out as offensive or defensive specialists, or both, but the mix of physical traits are what may be enticing in hoping for future development.
Cluster 6 -- Floor Spacing Wings
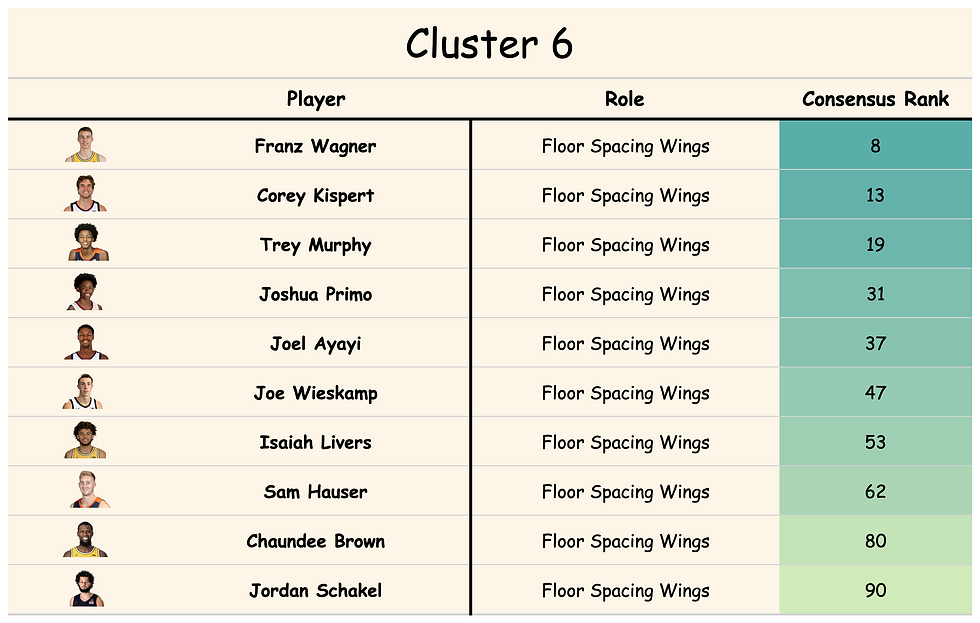
- Strengths
o 3PA/40, 3P%, 2P%, FT%, Height
- Weaknesses
o 2PA/40, FT/40, FTA/40, STL/40
Next, in cluster 6, we find our “connectors”. These are the guys who are “ceiling-raisers” and that are more than likely to contribute sooner than other prospects. They tend to be a bit taller/longer than most wings while also shooting lights out from the perimeter. Players like Franz Wagner, Corey Kispert, and Trey Murphy are guys that you might not expect to carry a large usage burden but are plug-and-play prospects who improve a team sooner than an upside swing. Duncan Robinson, the newly crowned 90 million dollar man, is the prototype of this role and with how the game has changed, don’t expect the importance of this cluster to change anytime soon.
Cluster 7 -- Attack Minded Guards

- Strength
o FTA/40, FT%, AST/40, 3P%, PTS/40
- Weaknesses
o Height, TOV/40, DRB/40, 2P%, BLK/40
Our next cluster of prospects is nothing short of the eye-popping athletes who attack the hoop at will. They get to the line more often than any cluster while also shooting a high percentage from 3. With an above-average AST/40 stat, primaries in college such as Mann, McBride, and Wright IV show that some are more than just scorers as well. While some of these names may be interchangeable with other clusters/roles, the relevance of this cluster has been a staple of the game.
Cluster 8 -- Big & Athletic Defenders

- Strengths
o ORB/40, STL/40, BLK/40, Height, Weight
- Weaknesses
o PTS/40, 3PA/40, 2PA/40, FTA/40
Cluster 8 is full of high energy guys who are absolute disruptors on the defensive end, especially with the first four names. Jones, Garuba, Lewis, and Pons are all athletic freaks who have made a name for themselves through this. On the other hand, their lack of apparent offensive skills is what keeps them from falling in other clusters. Below average in PTS/40, 3PA/40, 2PA/40, and FTA/40 show that they aren’t yet, or might never be capable of being a true asset on offense. This leads us to more questions about how teams should utilize their draft capital. Do you believe a specific prospect has the tools to add to his offensive game? How long do you think it will take for him to develop these skills? Can you wait that long in terms of front office pressure on winning? The more questions the better, but it goes to show how hard this evaluation process can be.
Cluster 9 -- High Usage Scoring Bigs
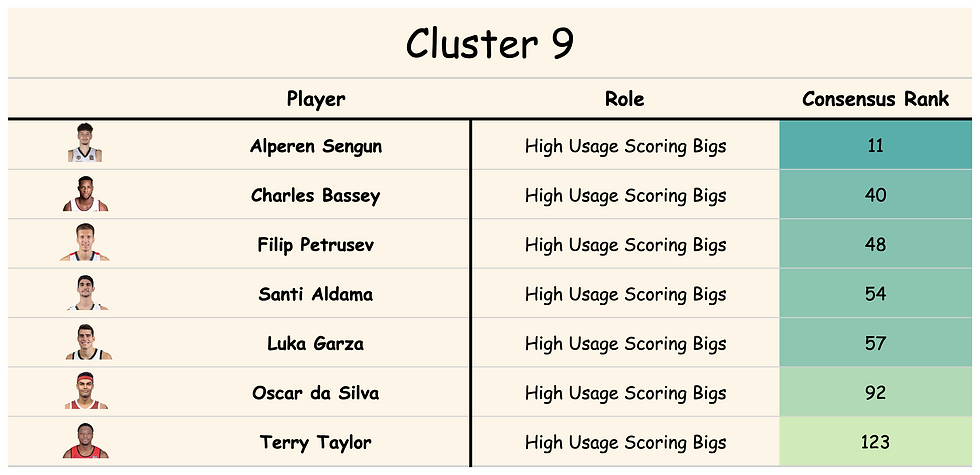
- Strengths
o FG/40, PTS/40, 2PA/40, ORB/40, FTA/40
- Weaknesses
o STL/40, AST/40, 3PA/40, FT%
Our 9th cluster is the group of throwback bigs who are the back-to-basket, I’m going to score every time down the court type of bigs in college. Players like Luka Garza and Alperen Sengun are guys that have, or would, terrorize college teams because of the lack of developed bigs across the country at their age. While most have dominated collegiately, they usually find it much harder to thrive at the next level. The centers in the NBA are mostly all freak athletes while also being taller and heavier than nearly any center they’d face in college. Realistically, there are far fewer spots for these prospects now, especially if they are non-shooters or do not possess otherworldly skills like our current MVP, Nikola Jokic does.
Cluster 10 -- Offensive Engine Guards
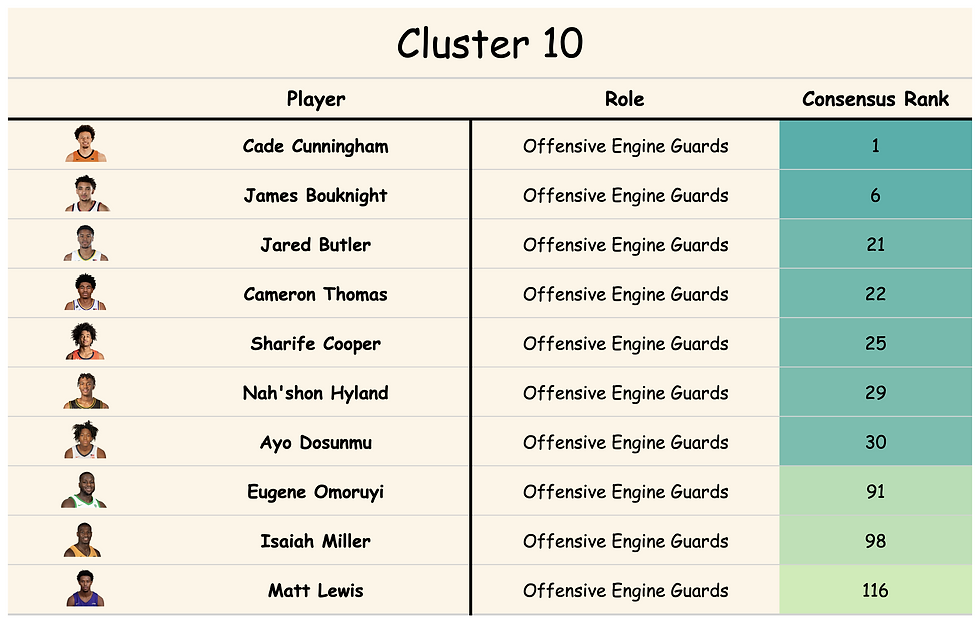
- Strengths
o FGA/40, PTS/40, FTA/40, STL/40, AST/40, Weight
- Weaknesses
o TOV/40, ORB/40, BLK/40, Height, Weight
Did our random clustering save the best group for last? With the first seven all being top 30 prospects, these are your do-it-all guys who are the difference makers on the offensive end. With above-average ratings in FGA/40, PTS/40, FTA/40, AST/40, and even STL/40, these high usage guys have become accustomed to leading teams. Whether or not they can replicate the same production in the NBA is a different question but being able to do it in college shows that they have at least developed some high-level skills that can translate immediately at the next level. Cunningham and Butler’s ball-handling and shooting mix, Bouknight’s scoring, Thomas’ and Hyland’s shot-making, and Cooper’s vision and passing are just a few that are evident.
With the player identification of the clusters wrapped up, I thought it would be interesting to see how the media has valued each specific role in terms of draft capital in the 2021 class. While there may be some noisy results, the gap from guards and wings to bigs and specialty defenders is massive and all but confirms what we are seeing with the modernization of the game.
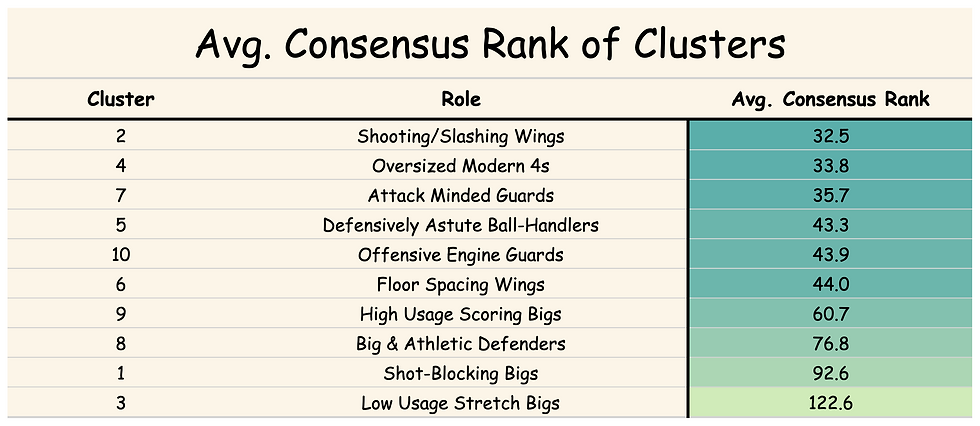
While these clusters/roles are crucial to success as a basketball organization today, the placement of players in such is completely subjective. Due to this, the average ranking may change a bit, but the long-term trends are apparent. Players who can score or shoot at a high level have a much better chance at sticking in the league today.
Limitations
While this investigation has been quite useful in identifying a potential alternative to the numerical ranking big boards, there are some limitations. The per 40 data used does not account for competition level (NCAA vs G-League vs International). Also, the data only uses the most recent season and ignores all prior information from past years. Not only that but some players are also tested on very small samples sizes, such as Sharife Cooper and Jalen Johnson, who participated in 12 and 13 games, respectively.
I touched on this earlier, but the clusters should not force us to pigeonhole players into permanent archetypes. These players are all unique talents who have the potential to grow into different roles. We should evaluate them for both what they are and what they can become in the future.
Conclusion
All in all, this study has certainly been both interesting and thought-provoking. The idea that all players fit the traditional positional labels is far outdated and new analyses methods such as clustering can offer a different perspective. Constructing positional big boards can help but an archetype-based approach can help improve our process of identifying which players fit specific roles. We should strive to acquire as much information and as many different views as possible on these widely disputed prospects. Asking more questions leads to more discussions, and ultimately better-informed decisions.
A final look:

If you are interested in similar studies, be sure to check these out:
- Ben Starks
- Alex Stern
コメント